IDE For LLMs
Build, Fine-tune and Deploy like never beforeThe Journey to Unearthing the Pain Points in Data Science Development
Zerve's mission to increase the impact of Data Scientists
Written by: Phily Hayes, January 30, 2024
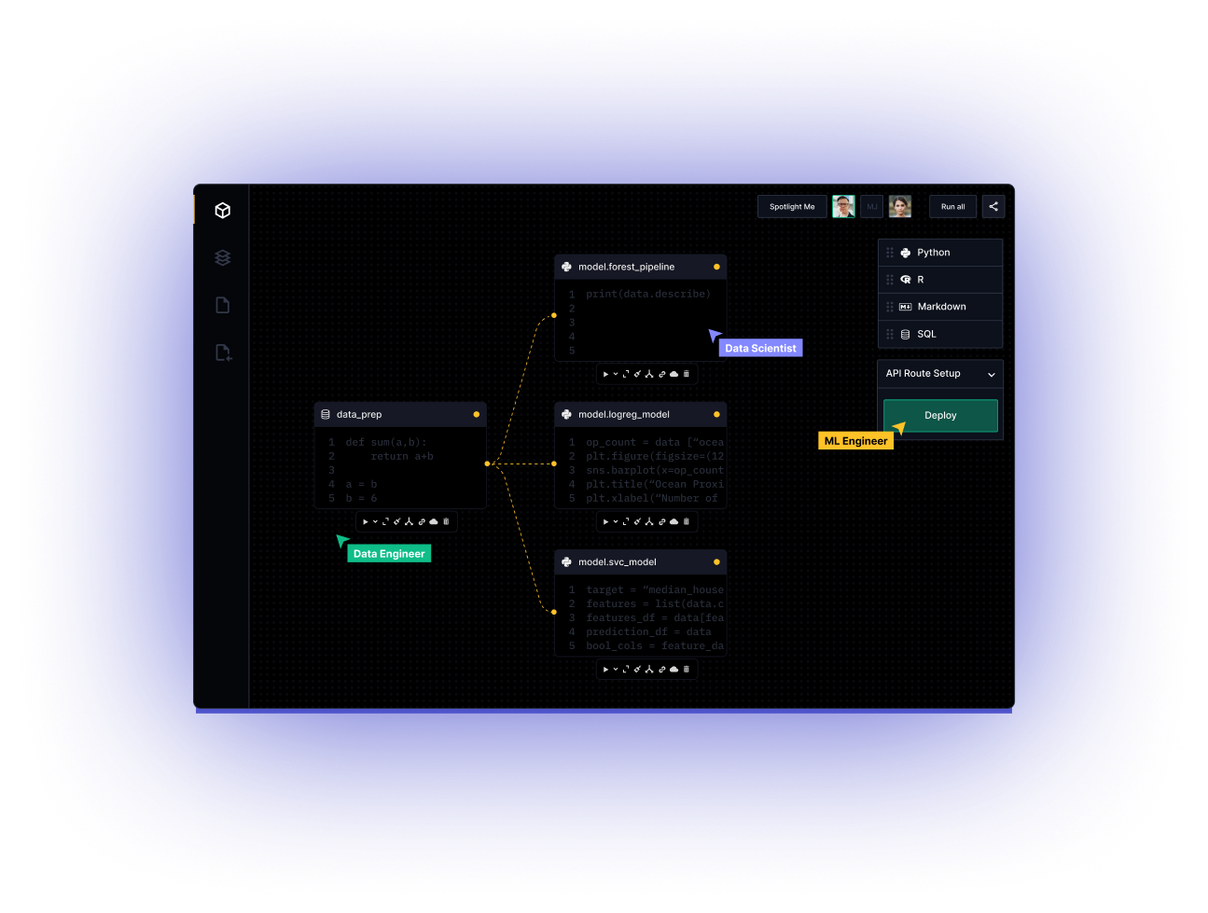
Content of the article:
- The Current Landscape
- Voices from the Field
- The Pain Points
- Getting Set Up
- Communication Challenges
- Deployment Hurdles
- Resource Configuration
- Demonstrating Work
- Leaders' Perspectives
- Enter Zerve
Share this article:
Our mission at Zerve AI is crystal clear: to Elevate The Impact of Data Science. This mission was born out of the frustrations our team had in delivering data science projects at scale, but also shaped by the community of “code first data users”, we have spoken to along the way (friendships were fostered!). The journey began with a commitment to understanding the daily challenges faced by those on the front lines of data—Data Scientists, Data Engineers, and ML Engineers. Zoom after zoom, we invested 100s of hours interviewing and getting to know every person. We knew our mission affected us, but having spent time with the communtiy, we knew it was something larger.
The Current Landscape
Our conversations created a sobering reality—the state of data science tools is hindering the full potential of truly exceptional people. The impact they should be making is stifled by the barriers erected by current solutions. Despite awesome inputs, too often limited outcomes were achieved by the individual or the team.
However, it's a bell curve, and of course there are representations not depicted in this blog, but it is the view we saw expressed most, otherwise we would not have dedicated ourselves to this mission.
Voices from the Field
Although we have a team of statisticians, we didn't just want statistics; we wanted stories. The anecdotes and quotes collected during our interviews brought life to the pain points experienced by our interviewees. It was a moment of shared frustration and laughter when we presented a slide encapsulating the daily struggles we had previously identified from interviews, as there tended to be a realization that their work-life was mirrored heavily by other folks in similar shoes around the world. I wanted to show you the exact slide I shared, (despite my web team telling me it's "ugly"!). Here it is, and like I mentioned, it often sparked laughter due to the resonation with the viewer (and maybe the design!).
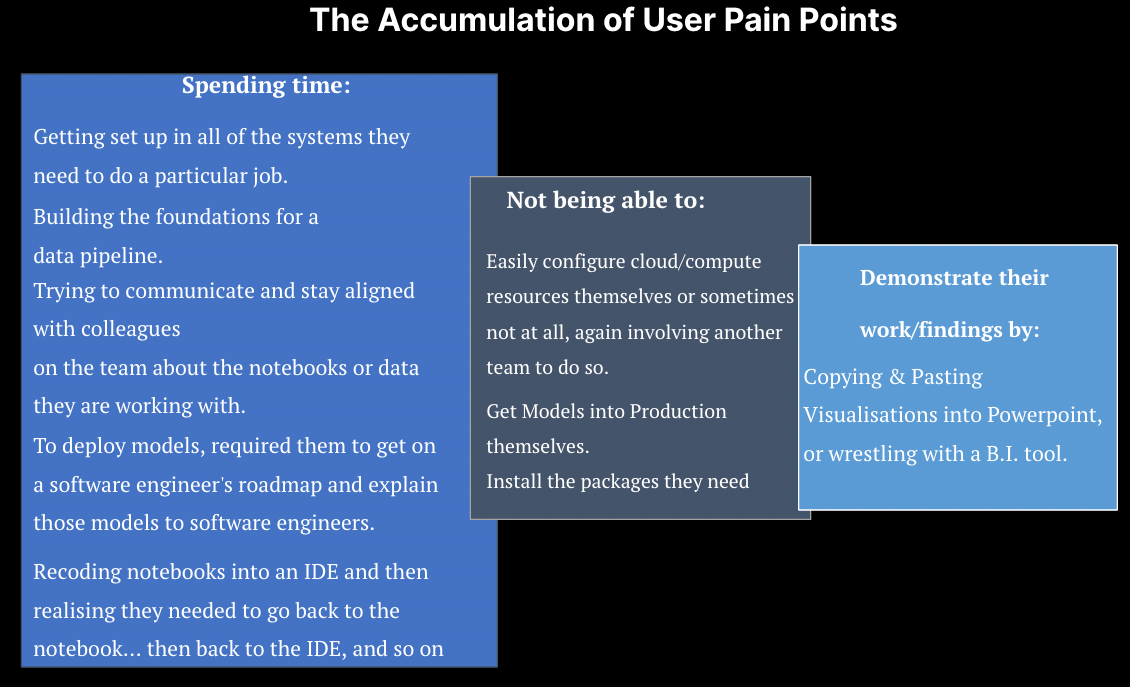
The Pain Points
Getting Set Up
One recurring theme echoed through the interviews was the substantial time spent getting set up in various systems at the beginning of an employment or at the start of a new project. Ensuring the right packages are used across a project was a constant pain point when it came to collaborating at exploration or handover stage. We heard of folks months into a job "ssh porting into a server to run a notebook" and describing it as "misery".
Communication Challenges
Aligning with colleagues about the intricacies of a notebook or the nuances of the data they're working with becomes a bottleneck, hindering collaboration and progress. We heard stories of people who have added daily meetings to ensure Notebooks are run in the right order, teams waiting to make progress on a project while a colleague was on leave due to local data or code, or a quite remarkable story where slack emojis were used to manage the statuses of an online notebook. Better processes exist of course, and these are not a reflection of teams we met, but the circumstances they found themselves in organisationally, when BAU often made it difficult to rectify the origin of the situation.
Deployment Hurdles
Deploying models is not a solo endeavor; it requires aligning with software engineers, often getting on their roadmap, and explaining the intricate details. What we felt from the interviews here, was an often awkward handover process. Depending on the type of organisation, the pain here could be felt on different levels, with frustrations occurring on both the engineering and data science side. Machine Learning, AI or Analytics Engineers are brilliant, but finite resources for working between the two disciplines.
Resource Configuration
Configuring cloud and compute resources shouldn't be a roadblock. However, for many, it is. The inability to easily configure these resources themselves—or sometimes not at all—adds layers of complexity, involving additional teams and further delays. They end up as a ticket for another team, and yet again not in complete control of the level of impact they can make.
Demonstrating Work
The struggle to demonstrate their findings is real. Whether it's the manual process of copying and pasting visualisations into PowerPoint or wrestling with a BI tool, the inefficiency in showcasing their work remains a significant pain point. The key problem here is that the lack of impact is felt across the board on this one. We had a CDO say “I thought I hired coders, but all I have 'gotten' is screenshots of charts in a PPT”. Of course the content of that presentation was all built with code, however the organisation had not given the team an effective way to share, via data apps, or integrations with core systems. It's just not where any organisation needs to be today.
In the next section, we'll explore how these pain points extend beyond individual experiences and affect the broader landscape of data science teams and their perceived value within organisations.
Leaders' Perspectives
The pain points identified in our interviews weren't isolated incidents. Leaders across organizations echoed a common sentiment: they weren't receiving the expected value from their data science teams. This sentiment reverberated through discussions with executives and managers who, time and time again, expressed dissatisfaction with the outcomes of their data science initiatives.
These leaders emphasized the gap between the immense talent within their data science teams and the tangible impact on business objectives. The disconnect was stark, with data scientists sometimes struggling to translate their high-level statistical work into solutions that could be efficiently integrated into existing workflows. This hit home really hard for the team at Zerve. The frustrations we felt coming back from the executive team was they had been misguided with their original hire, the data scientist. They were made to believe they originally hired something different to what they got, when in fact, the fault was organisational and there was not a road for delivering impactful results from the data scientist, and that’s why no impact was seen by the executive team.
Enter Zerve
Check out this post where we launch our solution in response to the information we received in the interviews above.
Published: January 30, 2024
Written by: Phily Hayes
Subscribe to our newsletter: